Uji Kinerja Sistem Denoising Sinyal Jantung atau EKG dengan Menggunakan Algoritma Empirical Mode Decomposition (EMD)
Performance Test of Heart Signal Denoising System or EKG Using Empirical Mode Decomposition (EMD) Algorithm
Abstract views: 337 | pdf downloads: 479
Abstract
Electrocardiograms (EKGs) are signals created by the electrical activity of the heart muscle and displayed on the EKG device's monitor. Using the EKG recording, the primary characteristics for diagnosing the status of the human heart can be determined. The death rate of heart patients can be reduced through early identification of cardiac problems. In ECG readings, it is frequently affected by several disruptions caused by muscle contractions and electrode movement. Numerous investigations on ECG signal denoising techniques have been undertaken earlier. This article examines the testing of the performance of EKGs using the denoising technique based on the Empirical Mode Decomposition (EMD) algorithm. In this work, many metrics were utilized to evaluate the ECG signal denoising technique: mean square error (MSE), mean absolute error (MAE), and signal-to-noise ratio (SNR). In this investigation, the ECG data was contaminated with noise from muscle artifacts (MA), additive Gaussian white noise (AWGN), electrode movement (EM), and baseline wander (BW). The noise-contaminated ECG signal is subsequently subjected to the denoising process. Calculate the MSE, MAE, and SNR values of the signal after it has been denoised. This study includes a scenario for testing three thresholding techniques with four distinct types of noise. The performance of the hard thresholding method is superior for all types of noise. MSE is produced by AWGN, which is 0.15, 0.28, and 9.9 dB. MA noise generates MSE, MAE, and SNR values of 0.4, 0.033, and 41.0 dB, respectively. The EM noise has an MSE of 0.010, an MAE of 0.04, and an SNR of 30.8 dB. The MSE produced by BW noise is 0.008; the MAE and SNR values were 0.0356 and 28.5, respectively.
References
Chai, T. dan Draxler, R.R. (2014) ‘Root mean square error (RMSE) or mean absolute error (MAE)’, Geoscientific Model Development Discussions, 7(1), hal. 1525–1534.
Clifford, G.D., Azuaje, F. dan McSharry, P. (2006) Advanced methods and tools for ECG data analysis. Artech house Boston [Cetak].
Donoho, D.L. (1995) ‘De-noising by soft-thresholding’, IEEE Transactions on Information Theory, 41(3), hal. 613–627.
Hamid, E., Das, S. dan Hirose, K. (2012) ‘Speech Enhancement Using EMD Based Adaptive Soft-Thresholding (EMD-ADT)’, International Journal of Signal Processing, Image Processing and Pattern Recognition, 5(2), hal. 1–16.
Jaenal, A. dan Usada, E. (2019) Elektronika Biomedik – Pembahasan Sinyal dan Citra Elektrokardiografi (EKG). 1st edn. Lentera [Cetak].
Joy, J., Peter, S. dan John, N. (2013) ‘Denoising using soft thresholding’, International Journal of Advanced Research in Electrical, Electronics and Instrumentation Engineering, 2(3), hal. 1027–1032.
Kuo, S.M., Lee, B.H. hal Tian, W. (2013) Real-time digital signal processing: fundamentals, implementations and applications. 3rd edn. United Kingdom: John Wiley & Sons [Cetak].
Mandala, S. dkk. (2017) ‘Performance analysis of wavelet-based denoising techniques for ECG signal’, in 2017 5th International Conference on Information and Communication Technology (ICoIC7). 2017 5th International Conference on Information and Communication Technology (ICoIC7), Melaka, Malaysia: IEEE, hal. 1–6.
Octaviani, E.S., Sari, Y. dan Suhandono, E. (2019) ‘Perancangan Aplikasi Konsultasi Online untuk Gangguan Jiwa Berbasis Web’, Jurnal Asiimetrik: Jurnal Ilmiah Rekayasa & Inovasi, 1(1), hal. 9–16.
Rahmasari, F., Wibowo, F.E. dan Pangestu, S.A. (2021) ‘Optimasi Penentuan Pemenang Pengadaan Tracker Antenna Menggunakan Metode Simple Additive Weighting (SAW)’, Jurnal Asiimetrik: Jurnal Ilmiah Rekayasa & Inovasi, 3(1), hal. 1–8.
Sahoo, S., Das, T. dan Sabut, S. (2016) ‘Adaptive thresholding based EMD for delineation of QRS complex in ECG signal analysis’, in 2016 International Conference on Wireless Communications, Signal Processing and Networking (WiSPNET). 2016 International Conference on Wireless Communications, Signal Processing and Networking (WiSPNET), Chennai, India: IEEE, hal. 500–504.
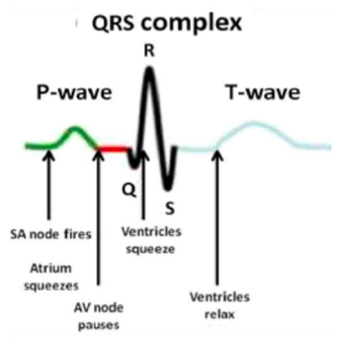